At Prospector, our machine learning and natural language processes (ML/NLP) help us monitor thousands of documents filed by publicly traded mining companies every day. The processes make recommendations on filing classification as well as data that can be extracted out of them to augment our database.
Our database already includes production data, mineral resource and reserve data, life of mine plans, and detailed ownership of each mine. High-confidence items are directly submitted to our database, while specialists review low-confidence items to help retrain models.
This human-in-the-loop approach allows our mining analysts to focus on complex data collection. Meanwhile, the artificial intelligence (AI) system constantly improves through specialist feedback and becomes more efficient over time, taking on more routine data collection tasks and enabling analysts to focus on high-value analysis.
As Google, Meta, OpenAI, Anthropic, and other large language model (LLM) development companies continue to push the bounds, we directly benefit from these improvements through our human-in-the-loop approach. As the first part of our AI series, I am going to take you through how we use our existing dataset with Anthropic's Claude2 model to drastically increase the speed at which we extract information from long 43-101, JORC, and SK-1300 technical reports. For this example, I am going to be using the Twin Hills project owned by Osino resources in Namibia.
-1.png?width=268&height=344&name=AI%20Blog%20Graphics%20(2)-1.png)
Let's start with the basics. We already know from our existing datasets that there is a completed preliminary economic assessment (PEA) and the maiden resource was announced in April 2021. We also know from some of our more basic ML/NLP models that this document is a 43-101 compliant Definitive Feasibility Study of the Twin Hills Gold Project. It was published on July 13, 2023 and it is 874 pages long!!! Wow...that is a lot.
Before Claude2, our analysts would open and analyze this report, identifying and calculating upwards of 50-70 metrics about this mining project. This includes details on overall life of mine plan, mining methods and metrics, processing methods and metrics, CAPEX, OPEX, and NPV assumptions. This process takes a typical analyst up to two hours depending on the complexity of the report. With Claude2's large token limit, we are able to send dozens of pages of text at a time and request the model’s recommendations based on specific prompts.
How do we start the process?
We cannot send the entire report. 874 pages is roughly 9-10 times the total limit, but we can isolate pages of text that we store in our database for specific analysis. First, we use the outputs of a query to identify the pages that most likely reference the mine life, minerals associated with the project, mining and processing methods, royalties, and projected start date. The total amount of pages of text usually ranges between 25-50 and is sent with our own prompt methods. In the case of Twin Hill, we received the following outputs:

What about the mineral resources and reserves?
These basic details are nice, but this is not a whole lot of information. We really want to know what resources and reserves are associated with this project. Similar to basics, we isolate the pages most likely to reference about mineral resources or reserves, which we send with a prompt to Claude2. For Twin Hills, we received the following response:

What about mining and processing?
The resources are more helpful than the basic details. But, we really need to dig deeper into the details to see if this mine will be profitable. Taking the same approach as before, we isolate the pages referencing the details of mining and recovery methods from the technical report. Sent with a prompt to Claude2, we received the following results related to the Twin Hills mine:
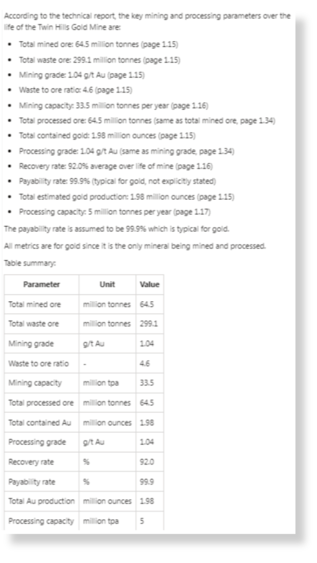
Show me the money!
But what does it cost? To estimate the net present value and internal rate of return we need to know what the pre-production capital expenditures (CAPEX), sustaining CAPEX, any planned expansion CAPEX, and closure CAPEX for the project. We also need to get a sense of the expected ongoing operational expenditures (OPEX) and how they relate to the mining, processing, and resource/reserve metrics we have already gotten. Finally, we need to know the input assumptions for overall business case of the mine (e.g. what is the price of gold, discount rate, tax rate, etc..). Taking the same approach, we then isolate the pages discussing the details of the CAPEX, OPEX, and assumptions discussed in the technical report. This is sent with a prompt again to Claude2 and the following results were received related the Twin Hills mine:
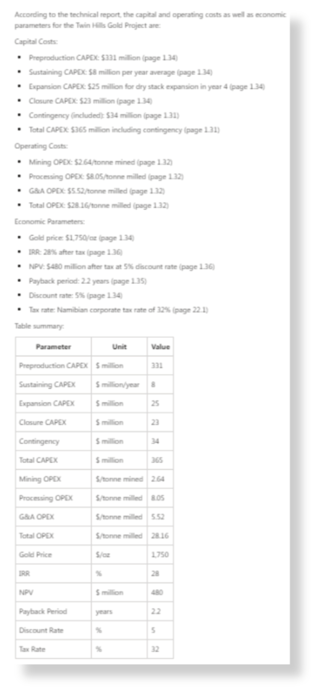
Now what?
With all these metrics, we now have all the basic details to recreate cash flow models and do basic NPV case analysis. Here, we look to Claude2 to save analyst time with an AI based analysis.
-1.png?width=400&height=609&name=AI%20Blog%20Graphics%20(1)-1.png)
In summary, large language models like Claude2 help us rapidly extract key data from technical reports, enabling faster database updates. This human and AI approach dramatically boosts our throughput so analysts can focus on high-value interpretation and analysis. As language models continue improving, our proprietary mining database will become even more efficient, granular and valuable.